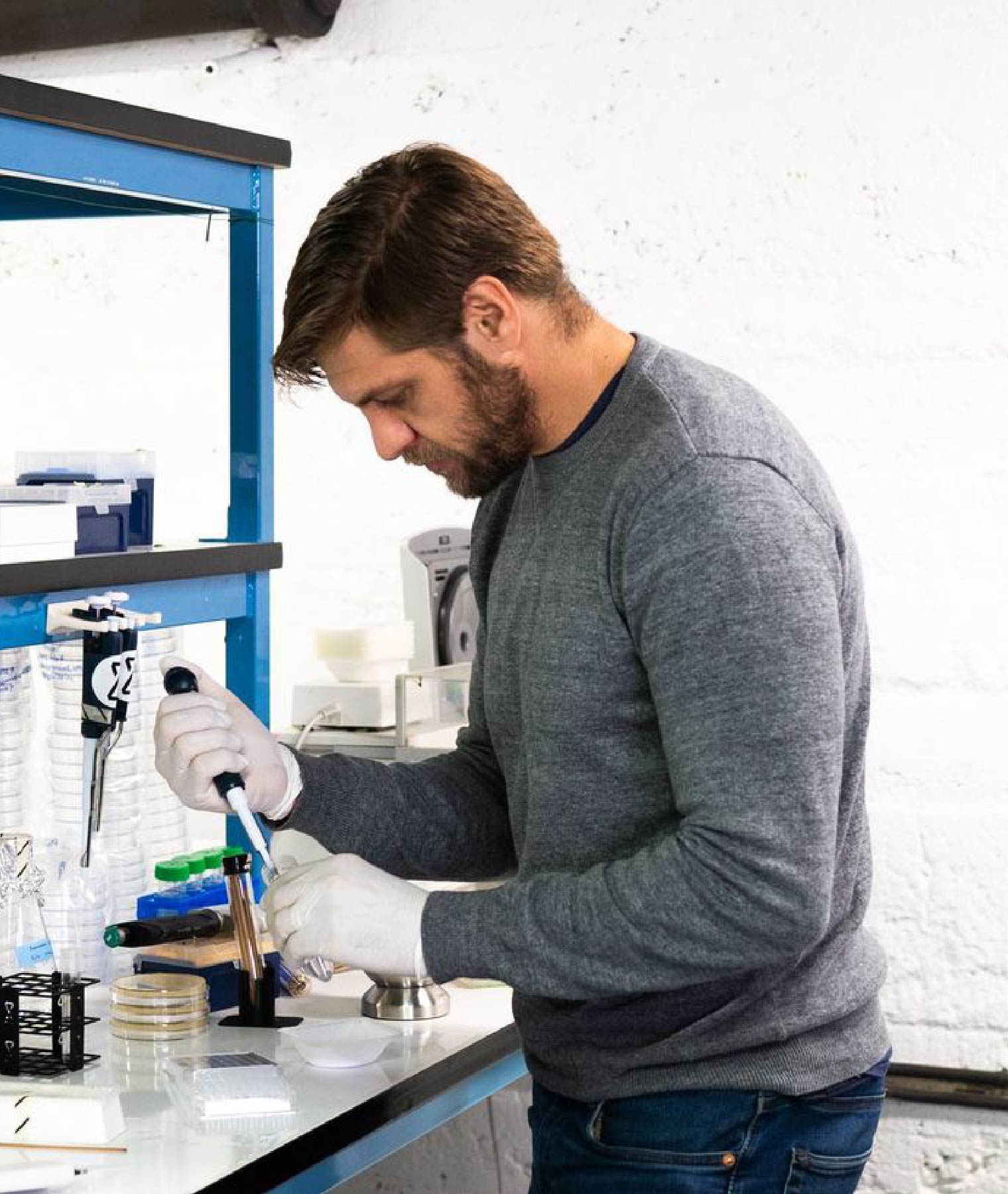
The internet makes information extremely accessible. Some of that information is good, and some is of course not so good. But how do we sift through it all, especially when information is so often presented as part of an author’s argument (and therefore is inherently biased)? While it’s a huge challenge, applying principles of scientific thought is a good place to start.
The current pandemic is an excellent illustration of this struggle to find truth in an online landscape dominated by politics and bias. What seems like the same scientific information is used to justify wildly different or constantly changing conclusions (e.g. Should we wear masks or not? Is sheltering helping or hurting? etc). How do we parse through it all in a way that makes us feel confident in our own decisions, without getting misled?
While scientists are human, and so of course have biases like anybody else, scientists are trained in tactics that help them avoid their biases as much as possible. As we all work through these challenging times, we are outlining some of these tactics here, with the goal of giving people a framework to parse through overwhelming amounts of information, tackle big personal questions, and feel more confident in their decisions.
Here are the four main strategies to help you think like a scientist:
- Be objective (acknowledge your own biases) →
- Look for the primary data (not the interpretation of that data) →
- Trust relevant experts (not people opining outside their area of expertise) →
- Be open to changing your mind (as new information becomes available) →

1. Be objective
Being human, we all have our own biases and logical fallacies. It is impossible to avoid them entirely. This is true for scientists too. But if we are aware of our biases and consciously try to overcome them, we become much better at finding the truth.
There are many things that can get in the way of our objectivity, but here are a few important ones to be aware of, many of which will show up in the examples in this article:
-
Confirmation bias: People more heavily favor information that confirms an existing belief
- To avoid this bias: Apply the same level of scrutiny to new information, regardless of whether it agrees or disagrees with your current position on a topic. For this bias specifically, a scientist is trained to try to disprove their hypothesis rather than prove it.
-
Dunning-Kruger effect: Experts understand complexity in their domain and are thus less confident at stating absolutes than people with more limited and simplistic knowledge in that same domain
- To avoid this bias: Do not necessarily equate the confidence of a person’s opinion with their level of understanding of the topic on which they are opining. Indeed, the opposite may often be true.
-
Belief bias: Once a person has cemented something as their “belief”, they will rationalize any piece of information to conform to that belief, rather than assess the true merits of an argument or new data.
- To avoid this bias: particularly on complex topics, do not assign absolutes to your opinions (e.g. what I believe is “right” or “true”), but rather think in terms of probabilities (e.g. what I believe is “90% likely” or “right, based on my current understanding”). Leave room in your mind for new information and understanding on the topic.
-
Group think: A person’s decisions and opinions are disproportionately influenced by the relatively small group of people they associate with, which can often be an echo chamber with social status penalties for dissent.
- To avoid this bias: Actively seek out alternative points of view (e.g. Google “arguments against shelter-in-place” if you and everyone you talk to are convinced that shelter-in-place is the right way to go), and try to read those points of view with an open mind.
-
Framing effect: People are more likely to accept an argument if it sounds or looks nice (e.g. the graphics on the webpage look high quality, the presenter is charismatic, etc.)
- To avoid this bias: While it can be almost impossible to ignore, try to strip away the bells and whistles and just focus on the substance of the argument and the actual relevant quality (or existence) of supporting data.
-
Halo effect: People are more likely to accept an argument from someone they like or find attractive (or reject an argument from someone they don’t like), or based on some other irrelevant aspect of their character (e.g. because they are a celebrity) rather than based on the merit or substance of the argument
- To avoid this bias: Ask yourself if you would accept/reject the same argument if presented to you anonymously.
-
Availability heuristic: People tend to weigh strange or recent information more heavily than information less easy to call to the front of their minds. Simply put, more available information has a disproportionate effect on our opinion, regardless of whether it is better information.
- To avoid this bias: Try to move beyond your immediate reaction and what first springs to mind, and search out an unbiased full picture of a situation, preferably with relevant primary data.
-
Backfire effect: Related to the belief bias, people dislike being “wrong” and thus can sometimes respond to even legitimate challenges to their core beliefs with a deeper retreat into and reinforcement of those beliefs.
- To avoid this bias: Approach discussions or contrary information with the goal of updating or nuancing your position, rather than defending it. If every conversation is all or nothing, you leave your brain no choice but to retreat in the face of a legitimate challenge rather than improve your viewpoint.
-
Irrelevant expert: People often over-value the opinion of an expert outside that expert’s area of expertise. For example, while seemingly related areas, a medical doctor is not by definition a virologist, any more than a plumber is an electrician.
- To avoid this bias: Consider that a title might be included as a framing effect, and ask yourself if this person’s expertise actually matters to this specific subject. For example, “Dr.” can be a dentist, psychiatrist, MD, PhD, etc. Dr. Smith, who is a psychiatrist, doesn’t have relevant expertise about viral infections. Therefore, you should apply as much scrutiny to Dr. Smith’s opinion about the value of wearing a mask for COVID as you would to your plumber’s opinion on the same subject.
Example: Acknowledging Our Own Biases
Consider a person who really wants shelter-in-place to end because they are going stir crazy. When they read an email saying that a primary care physician thinks shelter-in-place is causing more harm than good, the person may immediately accept it as valid and forward it on, all the while cementing in their own brain that shelter-in-place is wrong. However, a person thinking like a scientist would ask themself, “is this actually good information, or do I just want it to be?” Two issues at play in this example: confirmation bias and trusting an expert outside their area of expertise. A primary care physician is not an expert in epidemiology or population-level disease prevention, nor are they an expert in economics. So their opinion about shelter-in-place and its impact is no more valid than any other non-expert (more on this later).
A Note on Skepticism
An important point is that being objective is not just being skeptical or holding the default belief that new information is false until proven true. Immediately assuming something is not true is as unscientific as immediately assuming something is true. Objectivity requires taking in a piece of information with an open mind and searching for more information that either supports or refutes that information.

2. Look for the primary data
Ok so we’ve taken in information with an open mind and are working to be objective. Now we need to evaluate if the information is any good. For that, we look at the data on which the information is based.
When thinking like a scientist, we need to ideally find quality data from a primary source (i.e. a report of the data itself, not a secondary summary or interpretation of that data) that either is in support of or refutes the information in question. We also need to acknowledge when there is not enough data or the data available is not of a suitable quality to substantiate (or refute) the position. A scientist ideally only makes a decision when there is enough quality data to support a position. Otherwise, a scientist is forced to say “I don’t know enough to say” or “I don’t have enough data to say for certain, but here’s what I think based on X,Y, and Z.”
Example: Finding Primary Data
You read an article that says zinc is good for the immune system and that we should take it to prevent COVID-19. The article doesn’t cite any primary data, which makes you wonder if it is actually true, especially given that zinc is an accessible substance, and yet official health bodies like the NIH, CDC, and WHO are not broadcasting it as an important prevention measure alongside hand-washing, social distancing, and masks. So to validate or refute this, thinking like a scientist demands that we find some primary data on the subject to help us make a decision about whether to stock up on zinc supplements or dismiss the recommendation.
The first red flag for this specific article is that it is written by an expert from the wrong field (why is a transplant dietician writing about viral infections?) but we’ll get to relevant expertise in the next section. The second red flag is that nowhere in this article is there primary scientific literature cited. A good journalist will cite the scientific evidence. But that’s ok; it doesn’t mean it is necessarily not true. It just means we have to go out and find the data ourselves. So we are looking for data showing that someone tested zinc and showed scientifically that it has an effect.
A brief Pubmed search of “zinc and COVID” showed that there had been several studies (see analysis of multiple studies here) demonstrating that zinc supplementation might shorten the duration of the common cold, which is caused by a variety of different viruses that vary in their similarity and relevance to SARS-CoV-2. In addition, another study showed that zinc in conjunction with an ionophore (over-the-counter zinc lozenges do not contain ionophores, which allow the zinc to be taken up into cells) was able to inhibit SARS-CoV-1 RNA polymerases and reduce replication and cytotoxicity in cell cultures. SARS-CoV-1 and SARS-CoV-2 have very similar (95% identical) RNA polymerases (citation), so it is likely that the same is true for SARS-CoV-2. So, presented with this data, a scientist can reasonably conclude that zinc alone has been demonstrated to potentially shorten the duration of other viral infections, some of which have some relevance to SARS-CoV-2, and that it is likely that zinc – if transported into the cell with an ionophore – could inhibit vital virus machinery in SARS-CoV-2, given its effect on the closely related SARS-CoV-1.
Taken together, the primary data suggests that there is a somewhat reasonable hypothesis that zinc could help shorten the duration of a SARS-CoV-2 infection, but that no direct data exists to confirm that, and so it is difficult to say with certainty. While this conclusion may not sound satisfying (i.e. it is not “yes, take zinc” or “no, don’t waste your money”), it is also honest, and doesn’t overstate what we know and don’t know. Actions taken as a result of this conclusion can be done with a rational and clear head, and with plenty of room to amend practices in light of new data.

3. Trust relevant experts
We are trying to be objective and look for primary data to help us make up our minds on a topic, but often that is difficult or impossible, especially when a topic is very complex. When even interpreting data without an advanced degree in the relevant field is not possible, it is often necessary to seek out the opinion of an expert in that field. They are familiar with much more of the relevant research and are far better equipped to have a well-informed point of view on the topic. For this reason, when thinking like a scientist it is good practice to admit what you don’t know, and search for the opinions of a relevant expert.
However, it is important to note (as was discussed in another ZBiotics post), experts are only experts in their relevant area of expertise. Just as you wouldn’t ask chef Gordon Ramsey to weigh in on the importance of dark matter in the universe, you wouldn’t ask astrophysicist Neil De Grasse Tyson how to cook an incredible beef Wellington. This explains why a scientist wouldn’t blindly trust the transplant dietician in the example above as an expert on preventing viral infections; it’s completely outside their areas of expertise. If we want to know best practices on preventing viral infection and supporting the immune system, heed the advice of a virologist or an immunologist. And in the first example, rather than trust a primary care physician about shelter-in-place, seek out the opinion of an epidemiologist, biostatistician, and/or economist.

4. Be open to changing your mind
A scientist knows that “truth” is only as good as the current data. What we believe is true today is based on less information than we’ll have tomorrow, and much less information than we’ll have in a year. Therefore, stubbornly digging in your heels when faced with new information is as unscientific as making up your mind based on no information at all.
A scientist knows that changing your mind when new information is presented is not admitting that you were wrong, it is merely accepting that your previous understanding of the “truth” was the best you could do, given the relatively less information you had at the time.
For example, what is your best guess for what the picture below is probably a drawing of?
You’d say a circle, right? Of course. It almost certainly is, based on what we can see of the drawing. And while maybe one’s instinct is to say, “it is a circle”, thinking like a scientist would require us to have an open mind, acknowledging that we can’t see the whole picture yet, so we can’t say for sure: “Based on what we currently know, this is very likely a circle.” The media would likely then take that statement and say, “Scientists say drawing is of a circle”, because that’s basically what we said, right? Ok, but then when we reveal the whole drawing (i.e. when more data comes to light):
Now we know that it is actually a drawing of a keyhole. So now the media says, “scientists were wrong, it’s a keyhole, not a circle.” Obviously, we wouldn’t dig our heels in here and say, “I don’t care what that new data says, it is clearly a circle, based on previous data.” This example makes that response completely ridiculous. But all too often we make up our minds and then fail to revise our thinking as new information comes to light. A scientist knows that they weren’t wrong before in assessing that it was probably a circle, regardless of what the media said (they misquoted us anyway… we said it was “very likely a circle”). It probably was a circle, based on the information we had available. However, now we have better and more complete information, and so we must update our thinking accordingly.
A Note on Certainty
All too often in our society, conditional and indefinite answers are seen as evidence that someone “doesn’t know what they are talking about”, while absolute certainty is seen as a mark of the well-informed. However, interestingly and counterintuitively, these dichotomies should be viewed in quite the opposite lens; they are actually the result of the Dunning-Kruger Effect – another cognitive bias.
What occurs is the following: essentially, an expert will understand all the complexities and exceptions to the rules of the topic of their expertise, while someone with a very minimal understanding of the same topic typically sees things as very simple and straightforward. When faced with a seemingly simple question, therefore, an expert may couch their answer in conditional statements and acknowledged uncertainties, while the non-expert may answer with a direct and self-assured statement. Someone thinking like a scientist knows not to value the self-assured statement more than the expert opinion. They recognize that the more one knows about a topic, the more one realizes the astounding amount they don’t know. At the same time, they recognize that someone with a Twitter post-level of understanding of a topic might feel very confident stating bold conclusions, but that such conclusions are not the mark of being well-informed.
Thinking like a scientist requires us to recognize that many topics are complex, and that all too often only a preponderance of good quality primary data can provide clarity. Even then, good quality data rarely provides certainty; rather, it provides just a stronger and stronger probability of likelihood.
Put another way, when an idea is presented to someone thinking like a scientist, the probability of that idea being true is around 50% (i.e. as likely to be true as untrue). The higher quantity and quality of data presented in support of that idea, the higher the probability of the idea being true (or vice versa if data is presented in support of the antithesis). However, it would rarely rise to 100% certainty, as there is always a possibility (however unlikely) that new data comes out that does not fit perfectly with the idea, forcing a scientist to reevaluate their acceptance of the idea, at least in part.
Example: Changing Your Mind #1
In the early days of the pandemic, experts were telling us that face masks were unlikely to help reduce public risk of getting COVID-19, and that we should save the masks for healthcare professionals and other high-risk people. But that seemed contradictory: how can they not reduce our risk, while at the same time be needed to protect healthcare workers? Then the CDC came out and said that the public should be wearing masks to reduce the spread. At best, it seemed like no one knew what they were talking about, and at worst, it seemed like people were lying to the public.
However, thinking like a scientist, we now can understand this situation as one in which experts were making recommendations based on the best data available at the time. As new data came out, they updated recommendations by changing their minds, not by stubbornly sticking to their first opinion.
At first, we thought that the virus was not effectively transmitted by breath, and so masks were not necessary to reduce general public risk. However, because we did know that the virus was transmitted by fluid that could be coughed or sneezed out of an infected person, masks were known to be important for healthcare workers who were in close proximity to patients that were actively coughing (and effectively spewing virus) at them constantly. But then we got more information. Studies came out that demonstrated that even the breath of an infected person (even one that had not yet started showing symptoms) could carry lots of virus in very small droplets, and wearing any sort of barrier such as a cloth mask could reduce the amount that an infected person essentially breathed out the virus in a way that could infect others. Armed with this better understanding, the CDC (rather than digging in their heels and refusing to admit they were “wrong”) immediately warned the public and policy makers that widespread use of masks could help reduce transmission.
Example: Changing Your Mind #2
As a second, more personal example, I’ve long held the belief that GMOs are extremely important and valuable to humanity. I used to scoff at anti-GMO arguments that claimed they were somehow unsafe. However, I forced myself to challenge my biases, open up my mind, and explore whether there was something I was missing. Did I have all the information? Was I being too dismissive?
So I started spending a lot of time reading through opposition arguments to the use of GMOs. First and foremost, this exercise changed my own general view of the topic beyond a simplistic “these are 100% good” to a much more nuanced, “there are many benefits and great potential for this technology, but like with any technology, it comes with inherent risks and challenges that need to be carefully addressed.” Furthermore, I found interesting data that changed my perception of the probability of risk for GMOs. One example is this paper, which demonstrated that the genetic edit in Roundup-ready soy that makes a specific protein resistant to the herbicide Roundup (glyphosate) also causes that protein to tightly bind to the glyphosate, and results in detectible (although not dangerous) levels of glyphosate in the soy that we eat. This forced me to reassess my blanket belief that genetic engineering would cause no harm. While I don’t think this evidence suggests that GMO soy is harmful in this instance, it is a clear example of a nuance, a complexity, a complication of the issue beyond “good” or “bad” that comes with being better informed.
In Conclusion
Thinking like a scientist requires a person to gather unbiased information, lean on relevant experts, and be willing to update and change your position based on all the information available. By thinking like a scientist we can get closer to the truth.
In addition, thinking like a scientist has the added benefit of providing a framework to approach making decisions when faced with an overload of information. It asks that we search for primary data and the opinion of relevant experts, rather than trying to make sense of every bit of information out there. In a time when there is so much information available, it can feel overwhelming to even approach complex issues, but thinking like a scientist gives us the tools to start.